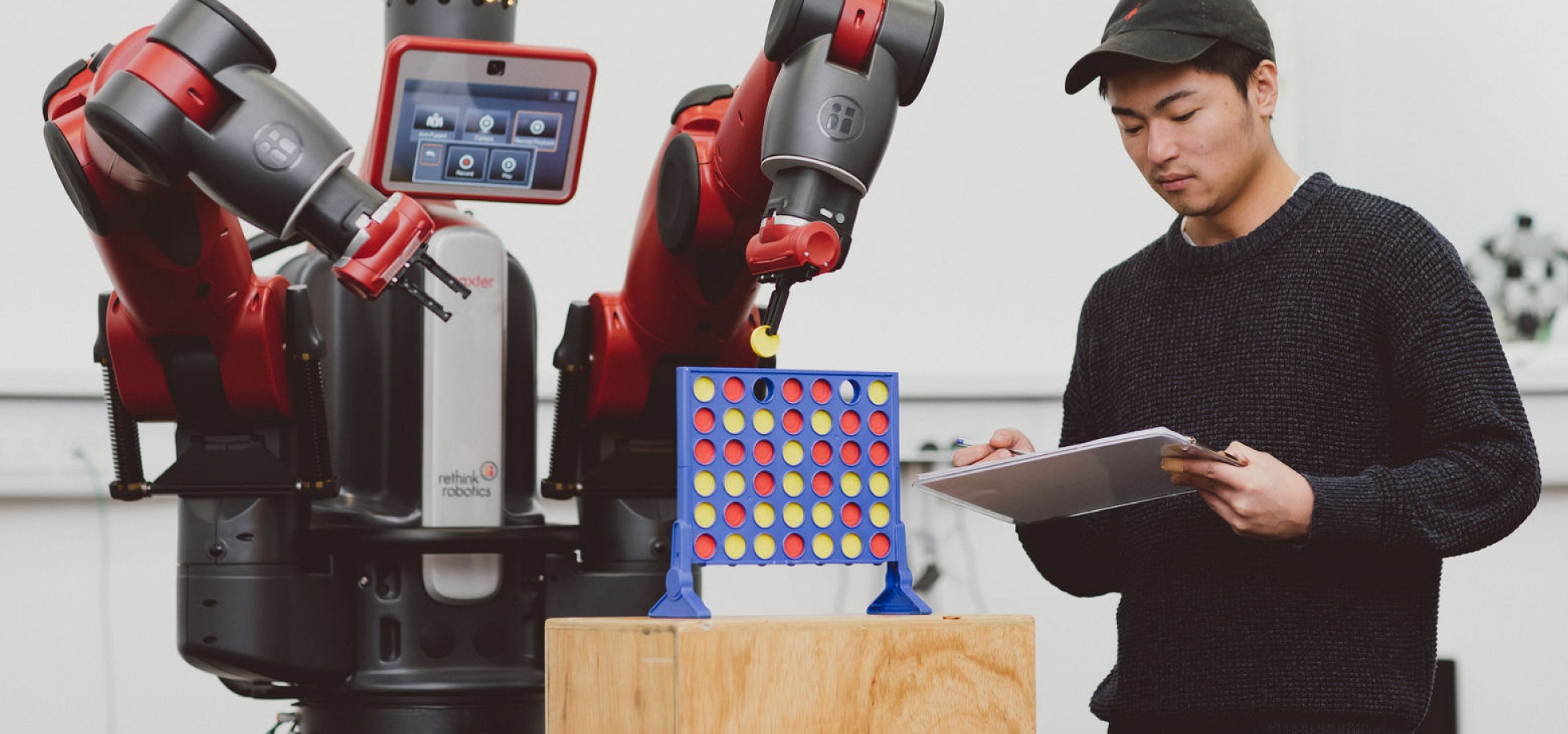
This module will introduce students to financial markets as well as providing a detailed introduction to the quantitative methods that are a pre-requisite to other CCFEA modules.
Students are introduced to financial markets such as equities, bonds, interest rates, forwards, futures and foreign exchange. Applications of calculus and statistical methods to finance are presented. Simple options (European calls and puts) are described, together with an introduction to stochastic methods and the Black-Scholes equation.
On completion students will have a knowledge of financial markets, a practical understanding of important quantitative methods, and knowledge of derivatives and the Black-Scholes Equation.
Students are introduced to financial markets such as equities, bonds, interest rates, forwards, futures and foreign exchange. Applications of calculus and statistical methods to finance are presented. Simple options (European calls and puts) are described, together with an introduction to stochastic methods and the Black-Scholes equation.
On completion students will have a knowledge of financial markets, a practical understanding of important quantitative methods, and knowledge of derivatives and the Black-Scholes Equation.
- Module Supervisor: Alexandros Voudouris
Module Description:
This module aims to introduce students to computational thinking in economics and finance by looking at different relevant models and theories, such as agent-based modelling and game theory. Students will also be introduced to various applications, such as financial forecasting, automated bargaining and mechanism design. Synergy will be explained i.e. students will be explained why some of the research in computation and finance or economics together can achieve something that they cannot achieve without the other.
Learning outcomes
By the end of this module, students will:
1) understand the basics of computational theories and modelling.
2) understand the synergy between computation and economics/finance
3) appreciate applications of computational thinking in finance and economics
This module aims to introduce students to computational thinking in economics and finance by looking at different relevant models and theories, such as agent-based modelling and game theory. Students will also be introduced to various applications, such as financial forecasting, automated bargaining and mechanism design. Synergy will be explained i.e. students will be explained why some of the research in computation and finance or economics together can achieve something that they cannot achieve without the other.
Learning outcomes
By the end of this module, students will:
1) understand the basics of computational theories and modelling.
2) understand the synergy between computation and economics/finance
3) appreciate applications of computational thinking in finance and economics
- Module Supervisor: Maria Kyropoulou
- Module Supervisor: Themistoklis Melissourgos
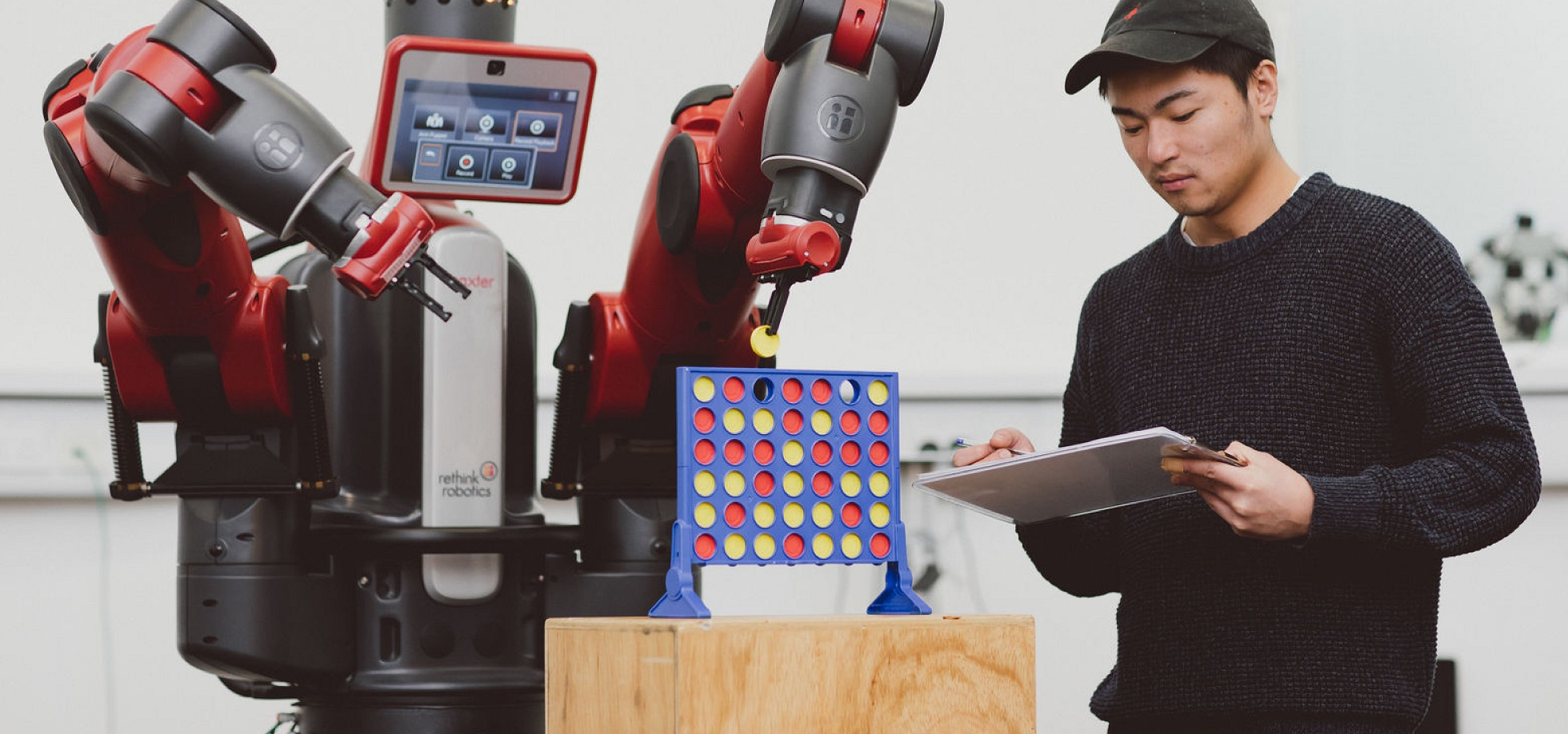
In this course taught in the Spring term, the student having obtained the prerequisites in traditional topics in derivatives and asset pricing in earlier modules taken, will progress to advanced topics involving models for risk management and derivatives pricing.
Topics here include Computational Methods in Option Pricing, Interest Rate Models, Credit Risk Models, and a brief introduction to machine learning in finance.
At the completion of this course, students will have acquired knowledge of cutting edge theories and computational skill to enable them to solve problems in a real world situation.
Topics here include Computational Methods in Option Pricing, Interest Rate Models, Credit Risk Models, and a brief introduction to machine learning in finance.
At the completion of this course, students will have acquired knowledge of cutting edge theories and computational skill to enable them to solve problems in a real world situation.
- Module Supervisor: Themistoklis Melissourgos
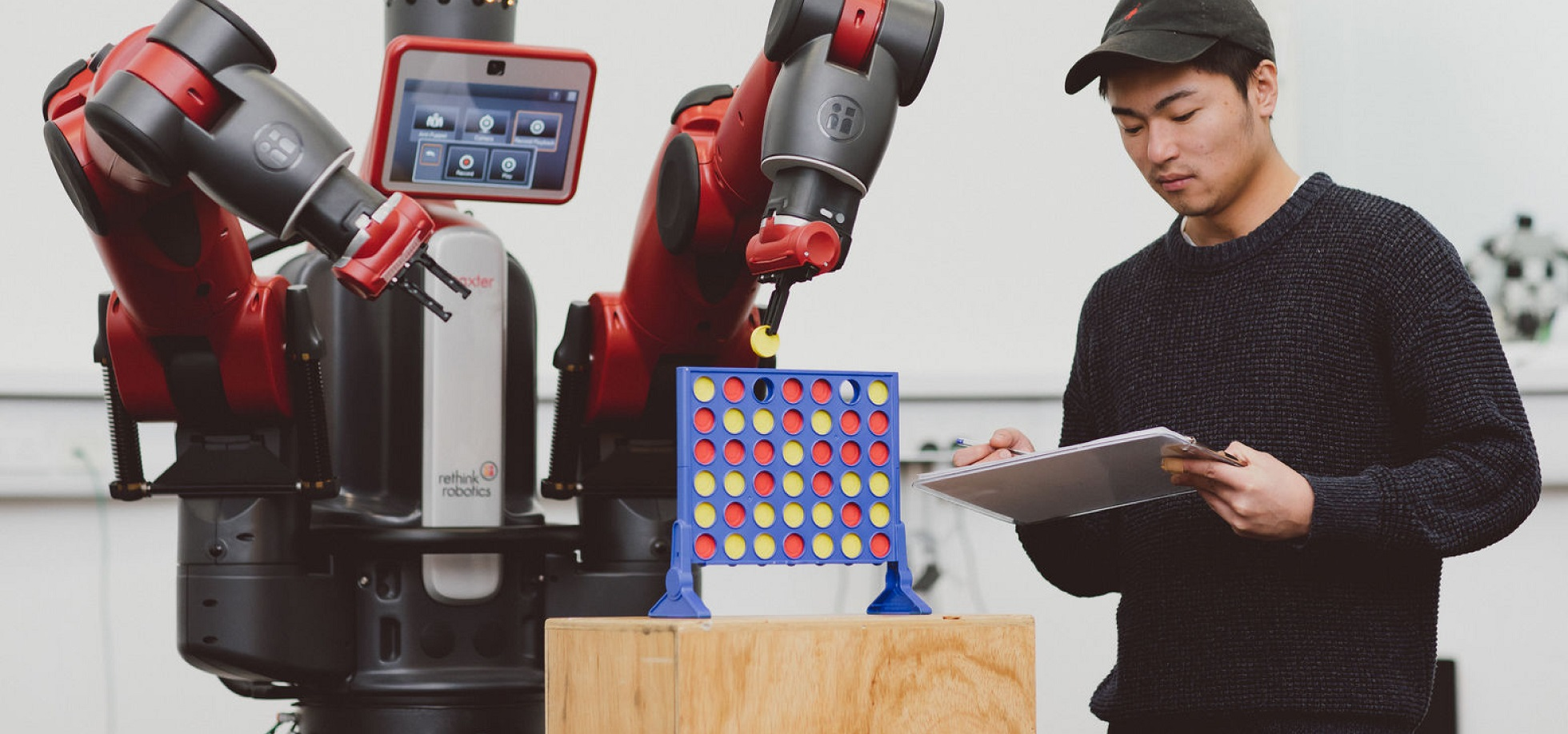
The vast proliferation of data and increasing technological complexities continue to transform the way industries operate and compete. Over the last two years, 90 percent of the data in the world has been created as a result of the creation of 2.5 exabytes of data on a daily basis. Commonly referred to as big data, this rapid growth and storage creates opportunities for collection, processing and analysis of structured and unstructured data.
Financial services, in particular, have widely adopted big data analytics to inform better investment decisions with consistent returns. In conjunction with big data, modern optimisation techniques (such as linear programming) are adopted to solve many computational finance problems ranging from asset allocation to risk management, from option pricing to model calibration. The continued adoption of big data will inevitably transform the landscape of financial services.
The module will be a mix of theory and practice with big data cases in finance.
For the theoretical part, the algorithmic and data science theories will be introduced and followed by a thorough introduction of data-driven algorithms for structured and unstructured data. Modern machine learning and data mining algorithms will be introduced with particular case studies on financial industry.
For the pratical part, the big data in finance cases will be introduced together with the study of relevant software tools.
Learning Outcomes:
After completing this module, students will be expected to be able to:
1) Understand the principles of (data-driven) algorithms such as modern machine learning and data mining algorithms
2) Understand the application of (data-driven) algorithms on financial industry
3) Use software tools to build up data-driven algorithms and analyse the huge amount of historical data
Financial services, in particular, have widely adopted big data analytics to inform better investment decisions with consistent returns. In conjunction with big data, modern optimisation techniques (such as linear programming) are adopted to solve many computational finance problems ranging from asset allocation to risk management, from option pricing to model calibration. The continued adoption of big data will inevitably transform the landscape of financial services.
The module will be a mix of theory and practice with big data cases in finance.
For the theoretical part, the algorithmic and data science theories will be introduced and followed by a thorough introduction of data-driven algorithms for structured and unstructured data. Modern machine learning and data mining algorithms will be introduced with particular case studies on financial industry.
For the pratical part, the big data in finance cases will be introduced together with the study of relevant software tools.
Learning Outcomes:
After completing this module, students will be expected to be able to:
1) Understand the principles of (data-driven) algorithms such as modern machine learning and data mining algorithms
2) Understand the application of (data-driven) algorithms on financial industry
3) Use software tools to build up data-driven algorithms and analyse the huge amount of historical data
- Module Supervisor: Panagiotis Kanellopoulos
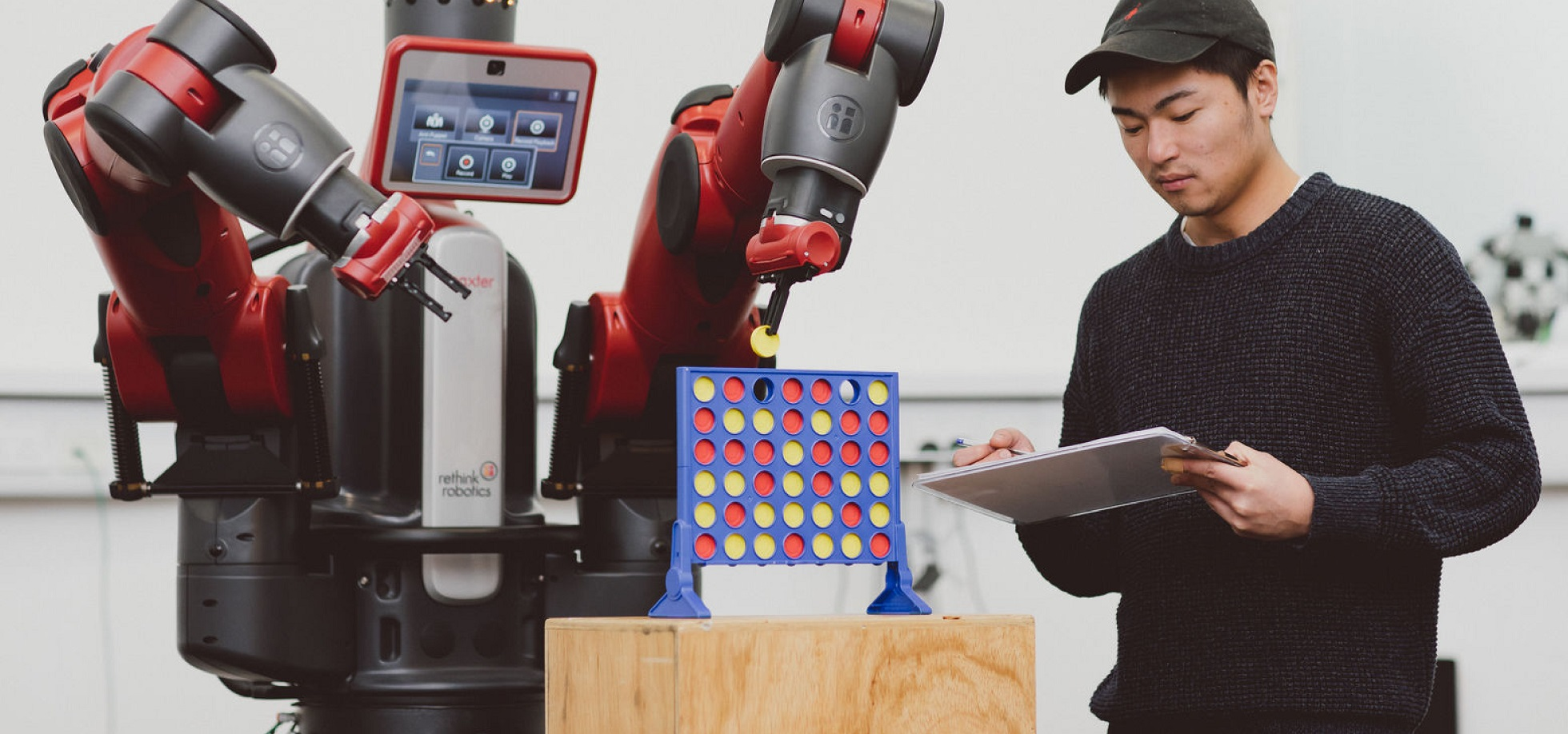
The vast proliferation of data and increasing technological complexities continue to transform the way industries operate and compete. Over the last two years, 90 percent of the data in the world has been created as a result of the creation of 2.5 exabytes of data on a daily basis. Commonly referred to as big data, this rapid growth and storage creates opportunities for collection, processing and analysis of structured and unstructured data.
Financial services, in particular, have widely adopted big data analytics to inform better investment decisions with consistent returns. In conjunction with big data, modern optimisation techniques (such as linear programming) are adopted to solve many computational finance problems ranging from asset allocation to risk management, from option pricing to model calibration. The continued adoption of big data will inevitably transform the landscape of financial services.
The module will be a mix of theory and practice with big data cases in finance.
For the theoretical part, the algorithmic and data science theories will be introduced and followed by a thorough introduction of data-driven algorithms for structured and unstructured data. Modern machine learning and data mining algorithms will be introduced with particular case studies on financial industry.
For the pratical part, the big data in finance cases will be introduced together with the study of relevant software tools.
Learning Outcomes:
After completing this module, students will be expected to be able to:
1) Understand the principles of (data-driven) algorithms such as modern machine learning and data mining algorithms
2) Understand the application of (data-driven) algorithms on financial industry
3) Use software tools to build up data-driven algorithms and analyse the huge amount of historical data
Financial services, in particular, have widely adopted big data analytics to inform better investment decisions with consistent returns. In conjunction with big data, modern optimisation techniques (such as linear programming) are adopted to solve many computational finance problems ranging from asset allocation to risk management, from option pricing to model calibration. The continued adoption of big data will inevitably transform the landscape of financial services.
The module will be a mix of theory and practice with big data cases in finance.
For the theoretical part, the algorithmic and data science theories will be introduced and followed by a thorough introduction of data-driven algorithms for structured and unstructured data. Modern machine learning and data mining algorithms will be introduced with particular case studies on financial industry.
For the pratical part, the big data in finance cases will be introduced together with the study of relevant software tools.
Learning Outcomes:
After completing this module, students will be expected to be able to:
1) Understand the principles of (data-driven) algorithms such as modern machine learning and data mining algorithms
2) Understand the application of (data-driven) algorithms on financial industry
3) Use software tools to build up data-driven algorithms and analyse the huge amount of historical data
- Module Supervisor: Panagiotis Kanellopoulos